7 Data-Driven Methods to Create Story Titles Using Keywords Analysis
7 Data-Driven Methods to Create Story Titles Using Keywords Analysis - Analyzing Search Intent Through Google Trends Data For Story Title Creation
Understanding the "why" behind searches, or search intent, using Google Trends is crucial for shaping story titles that connect with readers. Google Trends gives us a window into the ever-shifting landscape of popular searches, allowing writers to gauge current interests and tailor their content to match. This isn't just about spotting trending topics, but also understanding the specific nature of these searches. For example, are people looking for information on the fruit or the tech giant when they search "apple"?
By analyzing the data and identifying what people are genuinely curious about, writers can make smarter choices when crafting their titles. It's about leveraging data to guide the storytelling process, ensuring titles reflect what readers are currently engaged with. This method moves beyond guessing and aims to create titles that both capture attention and deliver on the reader's expectation based on their initial search. Google Trends provides a valuable springboard, helping writers translate current search trends into compelling story ideas.
1. By examining how search intent changes over time through Google Trends, we can see how public interest evolves in relation to current events, social trends, or even unexpected crises. This allows content creators to rapidly adapt and produce content that truly aligns with what people are actively looking for.
2. The data within Google Trends reveals how search patterns shift throughout the year. Recognizing these seasonal variations can help us craft story titles that are more relevant to a particular time of year, potentially boosting reader engagement.
3. Google Trends aggregates a huge volume of search queries, which can be useful for determining when a particular topic is at its peak popularity. This allows us to time the release of content strategically, aiming for periods when a topic is at its most engaging for the broadest audience.
4. Looking at search query data geographically, we can see where people are most interested in a given subject. This provides valuable information for crafting localized story titles that resonate better with readers in specific regions, improving the content's overall relevance and impact.
5. By analyzing related searches, we can get a sense of how conversations surrounding a topic are evolving. This helps in understanding new trends or shifts in the way people talk about a particular issue. This is helpful for creating titles that are both timely and interesting.
6. Google Trends has a feature that lets us compare different keywords. This can be used to understand which terms have the most search volume, informing us about what terms will generate the most attention. This is helpful for crafting titles that have a better chance of ranking highly in search results.
7. The trend data we get can reveal unexpected surges of interest in a topic. These spikes can point towards potential new stories, especially if they align with a creator's area of expertise.
8. Over time, we can track how people interact with the content we create based on the titles we use. This provides a valuable feedback loop for refining our strategies, allowing us to see which titles were the most successful in the past. This information informs better decision-making when creating future titles.
9. Trends that emerge through Google Trends can often reflect wider changes in society and politics. Recognizing this can help us stay informed about the issues people are concerned with. This allows us to craft story titles that reflect public sentiment and capture the attention of a broader audience.
10. While Google Trends is useful for understanding broader search trends, it may miss niche topics. This means that relying solely on Google Trends might lead to a narrow view of a subject. As such, it's important to combine the information from Google Trends with other types of research to ensure we have a comprehensive understanding of the subject matter we're covering.
7 Data-Driven Methods to Create Story Titles Using Keywords Analysis - Using Excel Pivot Tables To Extract Popular Keywords From Blog Comments
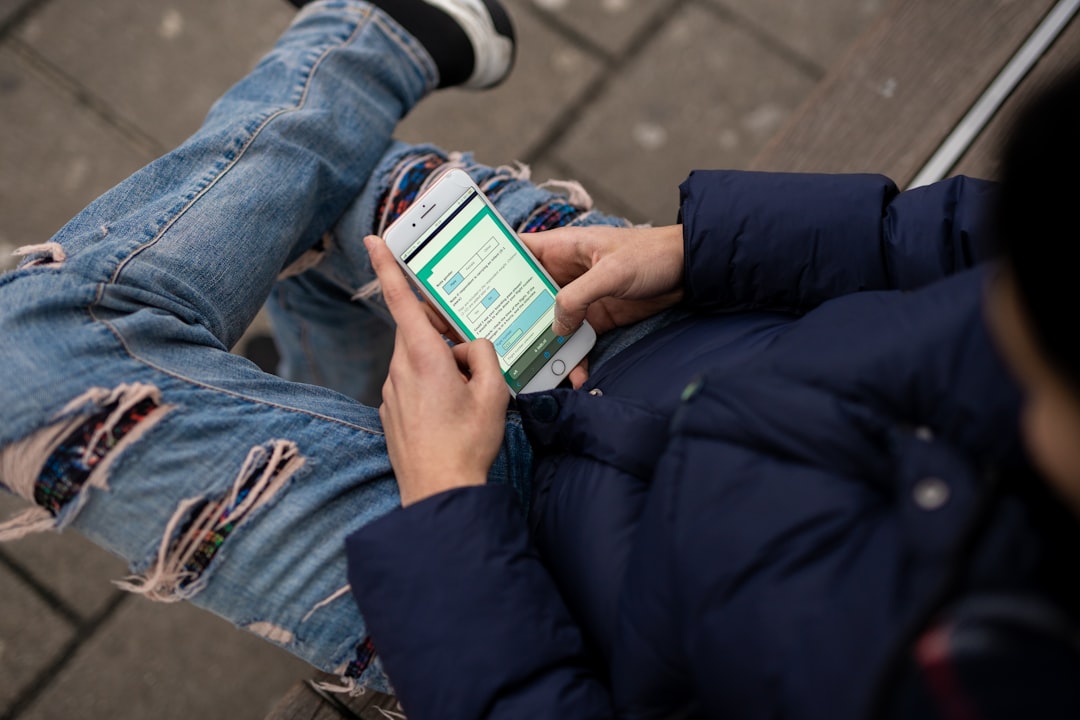
Analyzing blog comments for popular keywords can be a tedious task, but Excel Pivot Tables offer a powerful solution. These tables are designed to organize and summarize large datasets, which is ideal for processing the wealth of information found in comment sections.
Pivot Tables let you filter and sort the data in various ways, allowing you to easily isolate specific keywords. You can use filters to focus on particular sections of the comments or to pinpoint keywords that appear frequently. This process gives you a clear view of the language your audience uses, enabling you to understand what topics or phrases are most relevant to them.
Further, Pivot Tables can aggregate and count keyword appearances. This helps identify the most popular keywords within your comments, highlighting terms that are consistently used. Being able to quickly summarize and visualize this data makes it much easier to identify trends and patterns. This deeper understanding of reader engagement can inform the development of story titles that are more likely to resonate with your audience.
In essence, Pivot Tables transform the raw input of blog comments into actionable insights. By carefully utilizing their filtering and summarizing capabilities, you can extract meaningful data that can be applied to creating effective story titles.
Excel's Pivot Tables offer a potent way to dissect large datasets of blog comments and unearth the keywords driving reader interest. It's a powerful tool for summarizing and organizing data, transforming a jumble of comments into a structured view of the language used.
One notable aspect of Pivot Tables is their ability to filter and sort data, providing immense flexibility in analyzing keywords. Imagine filtering comments to isolate discussions about a particular product or issue. This granular approach enables writers to pinpoint precisely what's resonating with readers, providing a data-driven foundation for crafting relevant titles.
Further, Pivot Tables can handle aggregation and summarization, allowing you to count how many times a certain keyword appears within the dataset. This frequency analysis paints a picture of which keywords dominate the conversation, giving insights into the dominant themes of reader engagement.
Beyond simple counting, Pivot Tables can go deeper. They can be used to understand the sentiment related to certain keywords, whether it's generally positive or negative. This nuanced understanding informs writers on the emotional tone driving discussions, a crucial element in shaping titles that resonate with the current emotional landscape around a topic.
Pivot Tables can also show relationships between keywords and engagement. By pairing keyword usage with metrics like comment counts or likes, it reveals which keywords are fostering more active participation in the discussions. It's a valuable indicator for writers, steering them towards topics that naturally engage readers, leading to titles more likely to attract attention.
The time aspect of comments can also be woven into the analysis. With Pivot Tables, we can see how keyword usage evolves over time. This temporal perspective uncovers emerging trends and shifts in interest, guiding writers to craft titles that are not just relevant, but also timely.
If you have commenter data, it gets even more interesting. Pivot Tables can categorize keywords based on things like age, location or other demographic details. This allows writers to tailor story titles to specific segments of the audience, significantly increasing the chances of resonance.
Beyond simple keyword extraction, Pivot Tables can perform a type of thematic analysis. It can group comments based on shared themes and the keywords that appear within those themes, revealing hidden connections in the conversation. This kind of grouping can potentially suggest unique angles that wouldn't be obvious from a simple keyword count.
Pivot Tables are also adept at finding uncommon keywords. Imagine a keyword that unexpectedly spikes in frequency within the comment section. This could be a sign of sudden or emerging interest, potentially leading to story ideas that capitalize on a current event or change in public attention.
Furthermore, Pivot Tables are capable of identifying "long-tail" keywords. These are longer, more specific phrases that might be less frequent but indicate a very niche level of interest. These keywords can be gold for generating titles that target a smaller, but highly engaged audience.
Finally, Pivot Tables can help identify the 'key opinion leaders' among commenters. If certain commenters have high engagement and a unique vocabulary, their keywords can act as a beacon, indicating the core issues and perspectives driving discussion. Crafting titles that reflect these opinions can bring a sense of relevance and trustworthiness to the content.
Though Pivot Tables offer a lot, it's important to note that the quality of insights is dependent on the quality of the comment data. If the data is messy, incomplete, or suffers from biased sampling, the results of the Pivot Table analysis will be similarly skewed. Also, the insights derived from the Pivot Table should be used thoughtfully as part of a broader strategy, rather than as a replacement for actual understanding of the topic and target audience.
7 Data-Driven Methods to Create Story Titles Using Keywords Analysis - Implementing Python Scripts For Reddit Title Performance Analysis
Integrating Python scripts into Reddit title performance analysis offers a potent way to refine storytelling strategies. The Reddit API provides access to a wealth of data, allowing us to collect detailed information about title performance, including metrics like engagement and user interactions. Python scripts can optimize data retrieval through asynchronous methods, ensuring timely updates and access to real-time insights on what's trending.
Beyond simple data collection, these scripts can also incorporate data analysis methods like clustering. This helps us group subreddits based on commonalities in their content and user behavior, revealing patterns in what types of titles tend to perform well within those communities. By understanding the characteristics and preferences of different groups, content creators can tailor their title crafting to achieve better resonance.
Essentially, using Python to analyze Reddit data gives us a more nuanced understanding of the dynamics within these communities. This allows us to build a more accurate picture of what topics, formats, and styles of titles resonate with different user groups. The goal is to connect with readers more effectively, thereby increasing the chances that our stories find the right audience. This approach moves beyond trial-and-error and towards a more data-driven and strategic approach to crafting effective titles for Reddit.
1. Python scripts can be employed to carry out Exploratory Data Analysis (EDA) on Reddit data, specifically looking at things like upvote counts and comment numbers. This gives us a way to measure how well Reddit titles perform and see what kind of content really connects with people. We can then use this data to make changes in real time and get a better sense of what people in certain groups are interested in.
2. Python libraries can be used to perform network analysis of keywords found in Reddit titles. This analysis can help us uncover connections between different topics and themes, making it easier to create titles that feel more natural and connected to the discussion.
3. By looking at the performance of Reddit titles over time, Python scripts can identify recurring trends in how people engage with content. This lets us understand the lifespan of different topics and then use past successes to make better decisions when creating new titles.
4. Python is flexible enough to use data from many sources, like combining Reddit title performance with information from things like Google Trends. This kind of cross-platform analysis can give us a better overall understanding of how Reddit conversations fit into the larger online discussion.
5. Using natural language processing (NLP) libraries in Python, we can analyze the tone and emotional content of Reddit titles. This can have a big impact on how we craft titles because we can try to create a specific feeling or response in readers.
6. Python can automatically collect and analyze data from lots of different subreddits, providing a more complete picture of different types of audiences. This makes it easier to target our content towards specific groups, which is key for creating the most relevant titles.
7. We can use machine learning algorithms within Python to try and predict how well new titles will perform based on past data. This predictive modeling could become a useful tool for writers as they work on crafting attention-grabbing titles before they're even published.
8. Analyzing performance data lets us test different versions of a Reddit title using Python to streamline data collection and analysis. This type of experimentation is useful in isolating the components of a title that lead to the best results.
9. Python scripts can examine the relationship between the timing of a Reddit post and how it does in terms of engagement. This kind of analysis could lead to strategies for publishing content at times that will maximize the number of readers.
10. Using APIs and Python, we can access Reddit data in real time and immediately see how a title is doing. This gives content creators the ability to quickly change their approach based on how people are responding. This can really improve how relevant and interesting the content is over time.
7 Data-Driven Methods to Create Story Titles Using Keywords Analysis - Running A/B Tests On Social Media Headlines To Track Engagement Metrics
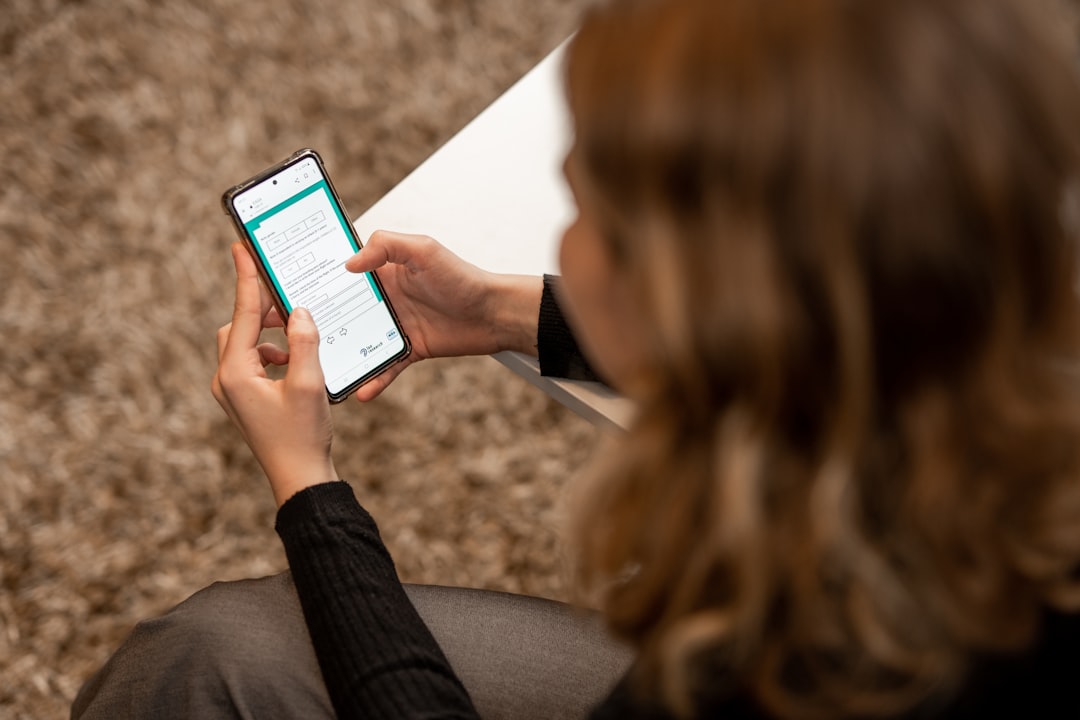
## Running A/B Tests On Social Media Headlines To Track Engagement Metrics
A/B testing headlines on social media offers a robust way to track engagement and glean insights into audience preferences. This involves creating multiple versions of a headline and showing them to different segments of your audience. By monitoring responses like likes, shares, comments, and clicks, marketers can identify which elements resonate most effectively. This approach is rooted in tried-and-true marketing techniques, offering a controlled environment for testing before broader campaign launches.
The value of A/B testing lies not only in its ability to capture real-time audience reactions but also in its potential to facilitate ongoing refinement. Successful elements discovered during testing can be incorporated into future content strategies, leading to continuous improvement. By leveraging this data-driven approach, content creators can tailor their social media output to better match the interests and engagement patterns of their target audience, leading to more compelling and impactful campaigns. Ultimately, A/B testing is about refining content, resulting in more effective social media strategies.
## Running A/B Tests On Social Media Headlines To Track Engagement Metrics
Examining the impact of subtle variations in social media headlines through A/B testing offers a powerful way to understand what drives reader engagement. By crafting two or more versions of a headline and deploying them to different segments of an audience, we can observe how changes in wording affect metrics like likes, shares, and comments. This approach allows us to move beyond guesswork and make data-driven choices about what resonates with specific audiences.
This methodology, derived from the practices of direct mail marketers, allows us to test the effectiveness of different approaches on a smaller scale before launching a full campaign. It provides a real-time feedback loop on audience preferences, enabling us to refine and optimize content for greater impact. This approach centers on understanding the nuances of how readers respond to different phrasing, visuals, and even the emotional tone of a headline.
We can gather crucial insights by meticulously monitoring key engagement metrics such as the number of clicks, the extent of reach, and how well the headlines contribute to specific goals, like driving conversions. Through rigorous data analysis, we can gain clarity on the degree to which the headlines are effective at encouraging desired behaviors.
The success of A/B tests on headlines can be leveraged for broader content strategy improvement. For example, incorporating elements from high-performing variations into future headline designs is a practical way to refine our approach. Additionally, it is important to establish clear objectives, utilize well-defined test groups, and meticulously analyze the data to extract useful and meaningful patterns.
Ultimately, this systematic testing approach offers a valuable method for understanding how audiences respond to different kinds of headlines. By tailoring our strategies based on insights gathered from A/B testing, we can improve the alignment between our social media content and audience expectations. This approach not only helps maximize audience engagement but also contributes to the creation of more effective marketing campaigns overall.
7 Data-Driven Methods to Create Story Titles Using Keywords Analysis - Creating Heat Maps From Website Analytics To Identify High Impact Words
Leveraging website analytics to generate heat maps is a valuable technique for pinpointing the most impactful words that drive user engagement. Heat maps essentially visualize how people interact with a web page, capturing clicks, scrolls, and areas ignored. This visual representation provides a clear picture of user behavior, revealing patterns and trends that can guide optimization efforts. The insights gleaned from these heat maps aren't just about improving website performance—they shift decision-making towards data. Instead of relying on assumptions, content creators can use heat map data to refine their story titles based on how people genuinely interact with a page. This continuous process of data collection and analysis allows writers to see patterns in engagement, which, in turn, empowers more informed adjustments to content. It's a method that, while powerful, is also accessible, as heat maps can be easily interpreted even by individuals with limited experience in data analysis. By understanding the "why" behind user interactions, content creators can use heat maps to increase the relevance of their story titles and, ultimately, improve the overall storytelling experience.
### Creating Heat Maps From Website Analytics To Identify High Impact Words
Heatmaps are a way to visualize how people interact with websites, providing a mix of numbers and visual cues. By showing where users click, scroll, and generally pay attention, they offer a window into how users experience a page or a product. Essentially, we're looking at where users' eyes are drawn to on a webpage, which can highlight the effectiveness of words and phrases used in the content.
These heatmaps can be incredibly useful for making website content better. Understanding where people focus helps us optimize layouts, making sure the most important information is seen first. Tools like Google Analytics and Mixpanel provide deeper insight into this user behavior, going beyond just clicks, to consider conversion rates as well.
But to get useful data, heatmaps require a decent amount of data collected over time. This can be a few days, maybe a week. Then, you can start to see patterns in how people interact, which will help make design decisions.
One thing that's interesting is the instant feedback heatmaps give. They provide a quick overview of website performance, flagging potential issues right away. They're also easy to use – even if you aren't an expert in data, you can interpret the results. This visual clarity is very helpful.
Some heatmaps are specifically designed for geographic data, using color gradients to highlight where certain things are happening. This can give insights into the demographics that are interacting with the website in a useful manner.
Ultimately, the visual aspect is extremely useful. You can easily identify problem areas and improve things based on the insights from the data. Instead of relying on our gut feeling about what works, we can use data to make more informed decisions. And, potentially, this can improve website performance and generate insights that lead to more effective strategies to boost conversions and engagement.
Furthermore, the use of heatmaps allows for a level of confidence when making decisions related to website performance. This data-driven approach helps guide decision-making and reduces reliance on assumptions. In some cases, this may lead to cost savings and a more effective use of marketing resources. It is worth noting that the insights derived from heatmaps are only as good as the data that they are created from, and as with any analytics endeavor, it's important to ensure the data is accurate and representative of the target population.
7 Data-Driven Methods to Create Story Titles Using Keywords Analysis - Tracking RSS Feed Performance With Custom SQL Queries
Analyzing RSS feed performance using custom SQL queries provides a powerful way to understand how well content is being delivered and consumed. By converting RSS feeds into a structured SQL database, you can analyze data without needing extensive programming skills, making in-depth analysis more accessible. This setup opens the door to tracking personalized performance metrics and tweaking the content strategy based on what the data reveals.
Furthermore, by crafting and running optimized SQL queries, you can accelerate data retrieval and processing. This speed is increasingly important for making sense of data in a rapidly changing online landscape. The ability to get these results quickly makes it possible to quickly adjust your approach as needed. This level of detailed analysis enables you to pinpoint which content resonates most with your audience and can help optimize your strategy across various channels, making custom SQL queries a valuable tool for maximizing RSS feed effectiveness. However, like any data analysis approach, relying too heavily on data can potentially obscure the bigger picture if the data collection and query design are not carefully considered.
1. Utilizing custom SQL queries to track RSS feed performance offers a granular approach to understanding how different content styles resonate with various reader segments. This allows for data-driven adjustments to content strategies, informed by distinct user behaviors. It's interesting to consider how we can translate these findings into improvements in content creation and delivery.
2. Custom SQL queries aren't just about monitoring engagement rates; they allow us to track the amount of time users spend interacting with specific RSS feed items. This detailed level of analysis helps us distinguish between content that keeps readers hooked and content they quickly skim over or ignore. It highlights the need to focus on quality and reader experience alongside the broader engagement metrics.
3. By combining custom SQL queries with data from other sources, it's possible to gain a broader perspective on user behavior across platforms. We can trace how RSS feeds drive traffic to other parts of a website or even to external sites, or how they influence conversions like newsletter sign-ups. Understanding this cross-platform relationship is crucial for maximizing the effectiveness of our RSS feeds.
4. Analyzing RSS feed performance using SQL can uncover unexpected trends, such as connections between specific keywords in feed titles and surges in user engagement. These insights could be valuable in fine-tuning content title strategies for better results, prompting questions about the degree to which specific keywords influence reader engagement.
5. SQL allows for a temporal analysis of RSS feed performance, which is helpful in determining whether certain topics see seasonal spikes in user interest. This type of analysis can help us strategically time the release of content to maximize audience engagement. It's thought-provoking how subtle changes in timing can dramatically affect user engagement with our content.
6. For more nuanced content analysis, custom SQL queries can differentiate user interactions based on demographic data, highlighting how various groups respond to the same content. This type of segmentation enables us to develop customized storytelling approaches catered to particular reader preferences. It's a reminder that our audiences are diverse, and our content needs to acknowledge and reflect that diversity for greater impact.
7. Monitoring user engagement metrics through SQL queries can provide a valuable feedback loop. This allows writers to adjust titles and content types based on the performance of RSS feed items over time. This empirical approach helps create more effective storytelling strategies that are rooted in actual user data rather than assumptions. The question becomes how to continually refine our strategies based on this ongoing feedback loop.
8. SQL queries can identify underperforming RSS feeds that might need content revamps or targeted marketing campaigns. This proactive approach can help maintain reader engagement over the long term, rather than relying on reactionary adjustments. The challenge lies in finding the balance between responding to feedback and not getting too bogged down in minor tweaks.
9. Integrating A/B testing capabilities within SQL queries allows for incremental improvements in RSS feed strategies, specifically identifying elements that drive success. This method goes beyond simple tracking and creates a culture of continuous growth and improvement. We could further investigate how to efficiently and ethically implement A/B testing within this context.
10. Custom SQL queries can unearth niche engagement patterns in RSS feeds that general analytics might miss, such as how specific linguistic styles or word choices affect user retention. This detail enriches content personalization efforts and strengthens connections with the audience. It's fascinating how the nuances of language can have a significant impact on audience engagement, highlighting the need for greater focus on linguistic choices and their impact on the reading experience.
7 Data-Driven Methods to Create Story Titles Using Keywords Analysis - Building Word Frequency Models Using Natural Language Processing Tools
Within the realm of natural language processing (NLP), building word frequency models is a fundamental method for extracting insights from written content. By analyzing the frequency of words within a text, we can uncover which terms are most prevalent and the overall themes emerging from the content. This analysis can be particularly useful for content creators who want to understand what their audience is interested in and adapt their writing accordingly.
Python libraries like NLTK and others provide readily available tools to automate and refine the process of word frequency analysis. These tools offer capabilities like tokenization (breaking down text into individual words or phrases), stemming (reducing words to their root form), and even sentiment analysis. This automation significantly speeds up the analysis process and reduces the possibility of human error in the analysis.
Moreover, techniques like generating word clouds or using N-grams can visualize the results of this analysis in an intuitive way. Word clouds are a great way to visually show the most frequent words and can help writers quickly identify the key topics within a body of text. Similarly, N-grams—sequences of words—provide insight into the co-occurrence of words, hinting at the potential relationships between concepts in the text. These tools can empower content creators to see the patterns and insights within their text, which helps guide them when crafting compelling and relevant story titles.
Ultimately, employing these NLP-driven word frequency models can provide a clear and actionable foundation for crafting more effective story titles. Writers are able to better understand the language and themes within their content and leverage these insights to create titles that directly connect with and capture the attention of the target audience, resulting in improved storytelling effectiveness. While these tools offer valuable benefits, there are also potential caveats, such as the need for large, high-quality datasets and the importance of interpreting the results within a broader context.
1. Understanding the emotional tone of text through word frequency analysis can be quite insightful. By focusing on the words that appear most often, we can start to grasp the overall sentiment of a piece of writing. This is crucial when crafting story titles that resonate with readers, as the emotional tone can play a big role in how a title is perceived.
2. NLP tools are incredibly useful for analyzing large amounts of text in real time. This means that we can continuously monitor how language is being used and quickly adapt to changes in reader interests. The ability to adapt to change can be a double-edged sword, as sometimes being responsive to short-term trends may not be the best approach.
3. Building frequency models can involve various linguistic features, like identifying parts of speech. This allows us to analyze not only which words are used but also how they're used within sentences. This deeper level of understanding can have a substantial impact on how we construct story titles, as it can reveal subtle differences in meaning and emphasis.
4. When we examine word frequencies in different contexts, we often discover unexpected relationships between words. Some terms may carry specific meanings or associations within certain genres or for particular audiences. This can be quite helpful in crafting targeted story titles, and also highlights the importance of considering target audiences when building these models.
5. In NLP, the process of tokenization can reveal details about lexical diversity. This is important for making sure that our titles aren't too repetitive, which can be off-putting to readers. Using frequency models, we can spot patterns of repetition and hopefully avoid making titles that are too monotonous.
6. Combining topic modeling and word frequency can be a powerful combination for unearthing hidden themes within a large body of text. This can be a great way to identify areas of reader interest, allowing writers to create titles that are more relevant and captivating. It's important to be mindful of how much detail we want to incorporate when topic modeling.
7. Word clouds, which are visualizations based on frequency models, are a straightforward way to see which terms are most common in a dataset. They provide a quick visual overview of trends without needing to dive into a complex analysis. This can be a nice way to have a first look at the data, but should not replace deeper analysis.
8. Frequency analysis can be combined with engagement metrics to see how well certain keywords are performing. This gives us a sense of how effective certain words are in attracting reader interaction. This kind of feedback loop helps us refine our title strategies based on how readers actually respond to different choices.
9. The language models we build can differ based on the datasets we use. This suggests that the same title might perform differently across various platforms or audience groups. It's crucial to keep these platform-specific nuances in mind when we craft our titles, which can present a complex challenge.
10. Although frequency modeling is a very powerful technique, it's critical to ensure that the dataset used to build the model is representative of the audience we are trying to reach. Using biased or unrepresentative data can lead to models that don't provide accurate insights. Careful consideration of the dataset is essential for gaining useful knowledge.
More Posts from storywriter.pro: