7 Science-Backed Methods to Evaluate Writing Prompts' Effectiveness in Fiction Writing
7 Science-Backed Methods to Evaluate Writing Prompts' Effectiveness in Fiction Writing - Measuring Word Count Distribution Patterns Across Different Prompt Types
Examining how word counts vary across different prompt types offers a window into how prompt design influences the volume of writing produced. For example, comparing prompts with varying time constraints, like those designed for 10 or 30 minutes, reveals noticeable differences in average word counts. This suggests that the amount of time given to compose a piece significantly impacts the writer's ability to generate text. Interestingly, some research hints that certain prompt formats, like those focused on descriptive writing, might lead to better results based on certain performance measures. However, it's important to recognize that these perceived improvements aren't always statistically substantial. The connection between prompt types and writing performance holds significance for both teachers and writers who are striving to optimize their writing techniques. The analysis emphasizes the complex interplay between prompt qualities and a writer's skill level, highlighting the necessity of careful consideration when designing writing prompts.
Investigating how word count patterns vary across different prompt types offers a window into the effectiveness of those prompts. For instance, a 10-minute writing prompt might yield an average of 147 words with a wide range of responses (standard deviation of 515), whereas a 30-minute prompt sees an increased average (308 words) with a tighter distribution (standard deviation of 126). This suggests that time constraints might be a significant factor in the length of creative writing pieces.
Interestingly, studies have explored whether the prompt's nature—descriptive versus narrative, for example—impacts the output. While some research indicates that descriptive prompts might lead to higher average scores, statistical significance hasn't always been demonstrated. It's worth noting that this area requires further investigation to solidify these findings.
Techniques like curriculum-based measurement (CBM) have been valuable in educational research for establishing standards across various writing prompts. These tools allow for a more objective assessment of the impact of different prompt structures on student writing. Studies on language learners, like those learning English as a foreign language (EFL), have shown that prompt type affects not just the overall word count but also other aspects of writing, including fluency, grammatical correctness, and vocabulary usage.
Moreover, meta-analyses of various writing studies have highlighted the effect of prompt type on things like syntactic complexity. These broad-scale examinations demonstrate that the way a prompt is framed influences how writers construct their sentences and paragraphs. However, the interpretation of writing quality, even in a standardized context, is prone to human bias. Studies have revealed that less experienced evaluators might be harsher than more experienced ones when scoring essays written based on particular prompt types. This introduces a significant layer of complexity when studying the effects of prompts on written output.
Some research groups have been looking at writing more holistically by using metrics like lexical diversity, recognizing that analyzing a range of aspects might give a more accurate picture of writing proficiency and fluency. Benchmarks based on these measures can be valuable tools for educators to gauge student progress and identify areas for improvement. It's clear that the type of writing prompt greatly influences the outcomes of writing tasks, affecting writing techniques, the consistency of evaluation, and the overall quality of writing.
7 Science-Backed Methods to Evaluate Writing Prompts' Effectiveness in Fiction Writing - Testing Reader Emotional Response Through Controlled A B Testing
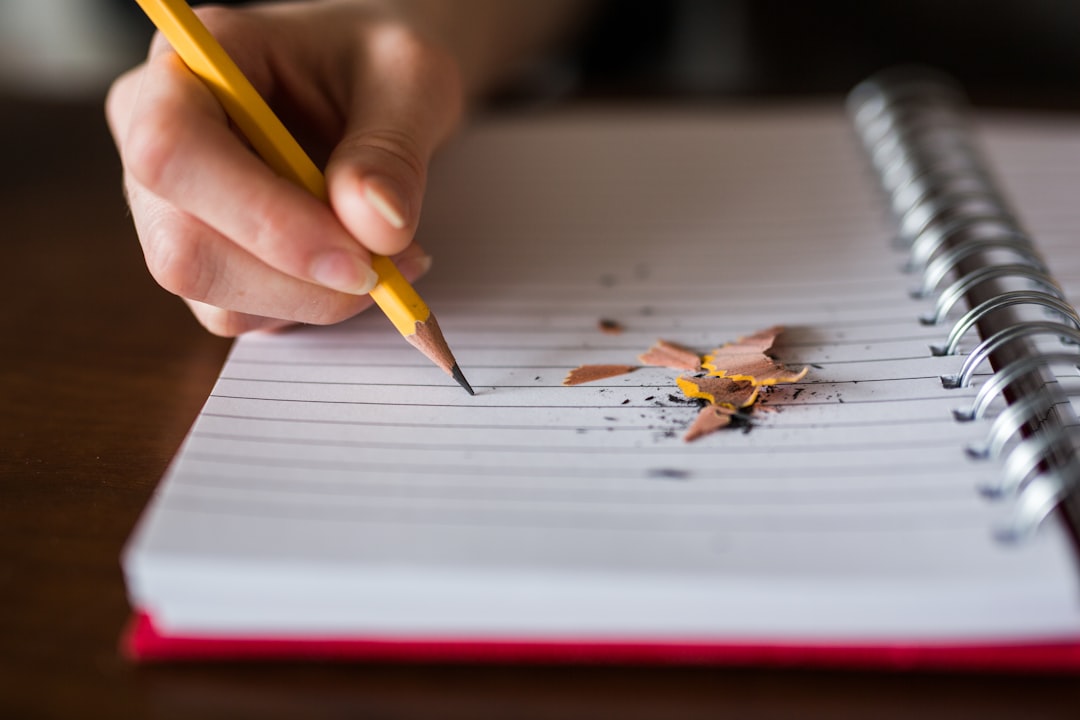
"Testing Reader Emotional Response Through Controlled A/B Testing" introduces a way to measure how writing prompts affect readers emotionally. It uses A/B testing, a process where two versions of a writing prompt are shown to different readers, to see which one creates a stronger emotional response and better understanding. The idea is that by carefully controlling the prompts and tracking things like how long people read and how they feel, writers can get a better sense of what works best in their storytelling. This method highlights the vital connection between emotions and reading, showing that understanding how readers react can improve a writer's work. It's important to be aware, however, of the ethical side of such tests, as influencing people's feelings can have significant consequences.
A/B testing can be applied to writing prompts to investigate how different phrasings or scenarios influence a reader's emotional engagement. We're interested in how various wording options lead to different emotional responses in readers, ultimately shaping their connection with the characters and plot.
Research suggests that emotional engagement, particularly when it's intense, plays a crucial role in how well a reader retains information from the narrative. This means that crafting prompts to elicit strong feelings could make the stories more memorable.
Examining the emotional language used in reader responses could potentially provide valuable insights into character relatability. By understanding the types of emotional words readers use, we might gain a better picture of how they perceive the protagonist and antagonist.
Intriguingly, a writer's own emotional reactions to a prompt can introduce bias and influence the outcome. If the writing process is clouded by a writer's personal feelings, it can affect the quality of the prompt itself and lead to less-effective writing.
Eye-tracking technology allows us to see how readers visually interact with text. Studies using this technology have shown that readers tend to dwell longer on passages with high emotional content. This finding implies that prompts that successfully evoke strong emotional responses could lead to more immersive reading experiences.
Interestingly, a study found that humor-based prompts might backfire if the humor doesn't match the overall tone of the story. This result emphasizes the importance of maintaining a cohesive emotional tone across prompts and the resulting narratives.
Researchers have experimented with using physiological indicators, like heart rate and skin conductivity, in A/B testing. The goal is to understand how different prompts impact a reader's level of anxiety or excitement, offering a more objective perspective on emotional impact.
It seems likely that different genres might react differently to emotionally-charged prompts. Horror prompts, for instance, might elicit more pronounced physiological reactions compared to romantic ones. This suggests that A/B testing needs to be tailored to each genre to maximize effectiveness.
Writers who engage in regular emotional A/B testing tend to find that the resulting reader feedback helps them understand which prompts work best. This type of testing not only refines their prompt development but also tends to improve their overall writing over time.
It's fascinating to consider that less traditional prompts, ones that evoke confusion or even a degree of discomfort, might actually result in higher levels of reader engagement and discussion. It seems there's a more complex relationship between inducing discomfort and stimulating interest than we might initially expect.
7 Science-Backed Methods to Evaluate Writing Prompts' Effectiveness in Fiction Writing - Analyzing Character Development Depth Using Natural Language Processing
Analyzing character development using Natural Language Processing (NLP) offers a novel approach to understanding how characters evolve and interact within a story. By employing advanced computational methods, writers can delve deeper into the intricacies of character arcs and narrative structures. NLP's ability to interpret and analyze language allows for a more nuanced evaluation of character interactions, emotional expressions, and the overall depth of character development.
Tools like Large Language Models are increasingly being used to not only improve writing quality, but also to assist in crafting more intricate and believable characters. This ability to move beyond basic grammar and style checks to understand narrative complexity is a significant development. This approach can greatly aid writers in refining their prompts to cultivate deeper character development. In this sense, NLP is not merely a tool for correction but a facilitator for understanding the intricacies of crafting engaging and relatable characters.
As NLP evolves, its capacity to aid writers in building richer character development and narrative structures is likely to expand. The promise of this emerging technology lies in its ability to enhance the storytelling process, allowing writers to create more impactful and memorable characters while ensuring narrative consistency and coherence. However, it's crucial to acknowledge that relying solely on computational analysis can potentially diminish the essential role of human intuition and creativity in the writing process. The future of this intersection between language and technology remains an area worth exploring with a critical eye.
Natural language processing (NLP) offers a way to delve into character development in a more objective way. By analyzing the language and dialogue used to portray characters, we can gain insights into the complexity of their growth throughout a story. This involves looking at things like the intricacies of language and how dialogue changes over time, giving us clues about how a character evolves.
We're seeing some fascinating developments with sentiment analysis within NLP tools, which suggests that characters' journeys might be viewed more favorably when a story maintains a consistent emotional tone. This has implications for reader engagement and overall satisfaction. It's as if a consistent emotional "thread" throughout the narrative makes the characters' development seem more coherent and appealing.
Word embeddings are a cool NLP technique where words are represented as points in a high-dimensional space based on their context within the text. Using this approach, researchers can map out character interactions and show how characters change over time. Sometimes, this method reveals surprising connections between characters that are hard to spot with traditional reading.
Studies show that characters described with a wide range of vocabulary, what's called lexical diversity, tend to create more engaging narratives. This suggests that using diverse and nuanced language can improve how relatable and appealing a character becomes. It's almost like a richer vocabulary helps readers connect with a character on a deeper level.
We can also apply network analysis to explore character relationships. This allows researchers to pinpoint social structures within the story, revealing how the connections between characters develop and change. This type of analysis can provide a clear view of how deeply a character is intertwined with the plot.
NLP, when integrated with character analysis, helps highlight discrepancies or inconsistencies in character development. For instance, we might find that a character's motivations or actions don't align throughout the story. These types of inconsistencies can decrease the quality of the narrative and make it harder for readers to truly immerse themselves in the story.
Interestingly, analysis of dialogue through NLP shows that characters with unique ways of speaking or specific vocabularies tend to feel more well-developed. This suggests that a writer's stylistic choices, even at the level of language, contribute to how readers perceive character depth.
Furthermore, NLP can help identify repeating themes or patterns in how characters develop. Writers can see if the ways their characters change over time align with familiar storytelling patterns or tropes. This insight can inform future writing decisions.
Research indicates that NLP-based metrics, such as how well readers stay engaged with the text and whether they come back to it, are strongly connected to the emotional depth of the characters. Characters with greater emotional depth tend to lead to a better understanding of the narrative.
It's possible that NLP tools could be developed to help writers create characters that better resonate with readers' expectations. This could improve the connection and empathy readers experience, which are important elements for effective storytelling. We're still in early stages of figuring this out, but the potential for NLP to bridge the gap between writers and readers is really intriguing.
7 Science-Backed Methods to Evaluate Writing Prompts' Effectiveness in Fiction Writing - Tracking Plot Coherence Through Automated Story Structure Analysis
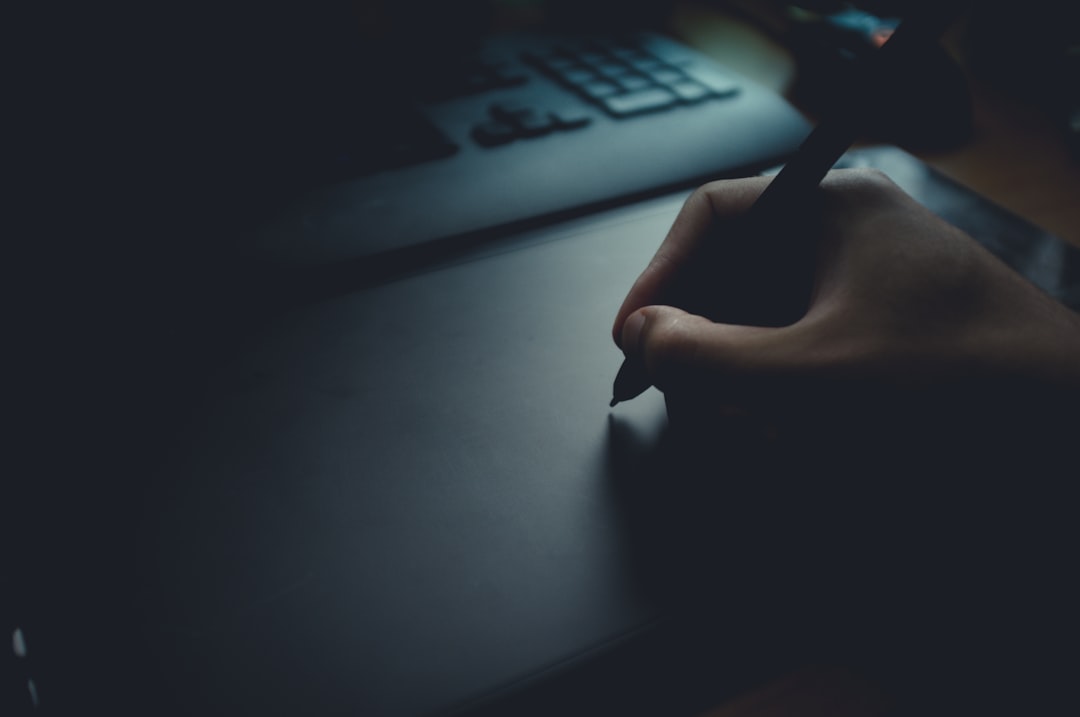
Analyzing plot coherence through automated means offers a new way to understand how effective a story's structure is in fiction writing. Using techniques that measure how often certain language elements appear—like pronouns and helper verbs—writers can gain insights into the story's overall coherence and how well its parts connect. Powerful algorithms can be used to explore massive collections of stories, allowing us to see core narrative structures, like the classic Freytag's Pyramid, and how plot events are linked together in a cause-and-effect way. While AI tools can suggest plot ideas, they need human intervention to ensure the story is clear and emotionally impactful. As this technology develops, its potential to aid writers in constructing well-structured and compelling stories grows, but it also raises concerns about the interplay between automated processes and the essential element of human creativity in storytelling.
We're starting to see how computers can help us understand the nuts and bolts of good storytelling, specifically when it comes to plot coherence. It turns out that tools that use machine learning can actually predict if a story's plot is well-structured, allowing authors to get a quick sense of where the plot might be strong or weak without having to do a deep manual dive.
Researchers have found that many popular stories follow certain classic plot patterns, like the well-known "Hero's Journey." Automated systems can measure how close a story sticks to these templates, which can give us another way to assess plot coherence. Interestingly, these systems can also use math-based models to analyze how often certain plot elements show up and how they're spread throughout the story. This gives writers a way to see if their story deviates from typical patterns in a quantifiable way.
It seems that keeping readers emotionally invested is a key part of building a coherent plot. Tools are emerging that can trace the emotional arc of a story, showing whether it keeps a steady pace and level of tension. This sheds light on whether the story keeps readers engaged throughout. It's not just about plot, though. These algorithms can also examine character development alongside plot coherence, uncovering whether a character's choices make sense within the overall story. If a character does something that's totally out of left field, these tools might help authors catch it.
There are now sophisticated algorithms designed to pinpoint how well different parts of the story connect to each other. Think of it as the story's internal glue. Higher "cohesion" scores mean that readers tend to grasp the story better and stick with it. This offers some pretty convincing proof that we can objectively assess these relationships. Researchers using automated analysis tools have found that stories with a moderately complex plot tend to be easier for readers to remember. It's a delicate balance, though. It's important to find that sweet spot between too simplistic and overly complicated to enhance the storytelling experience.
Interestingly, authors can even use these tools to compare their work to other successful stories, giving them a sense of what techniques led to strong plot coherence in established works. This could be a useful resource for newer authors to explore. The field is moving even further, with technology that can make dynamic adjustments to plot outlines based on assessments of coherence. This is a very exciting area because it might allow writers to actively refine and strengthen their stories in real-time, improving the overall clarity and reader experience without sacrificing their creative spark.
However, it's crucial to keep in mind the potential downside. Relying too heavily on these analytical tools might lead authors to inadvertently stifle their individuality or creativity. There's a danger that writers could try too hard to conform to algorithms instead of exploring more original paths. It's a reminder that while these tools offer a new lens through which to view plot coherence, they should be used as a supplement to, and not a replacement for, human intuition and creative flair.
7 Science-Backed Methods to Evaluate Writing Prompts' Effectiveness in Fiction Writing - Evaluating Genre Consistency Using Machine Learning Algorithms
**Evaluating Genre Consistency Using Machine Learning Algorithms**
Using machine learning to assess how well a piece of writing fits within a specific genre is an intriguing way to understand the patterns and characteristics that define different genres in fiction. Machine learning methods, such as those based on analyzing the relationships between words in sentences (syntactic dependency tree n-grams), can help writers identify linguistic features that are common to certain genres. This can give them a better understanding of how to achieve consistency in their writing style.
However, there are potential pitfalls to be mindful of. Over-reliance on automated methods for analyzing genre can be problematic, potentially leading to a simplified view of the complexities of writing or overly rigid classifications of different genres. The artistic nuances of creative writing often transcend strict categorization. While machine learning can prove to be a helpful tool, it should not overshadow the important role of human judgment and the writer's individual understanding of the craft of writing. In the end, machine learning can provide valuable insights for genre analysis, but it's most effective when it's used in conjunction with a deeper understanding of writing and style.
Machine learning, particularly in its application to natural language processing, has emerged as a promising method for examining the consistency of a piece of writing within a specific genre. By training algorithms on a broad range of writing samples that represent diverse genres, researchers can develop models that recognize subtle patterns in language and style. These patterns can include sentence structures, vocabulary choices, and even the use of punctuation, all of which can be indicative of a particular genre.
For instance, romance fiction often uses more emotionally charged vocabulary, while thriller writing tends towards shorter, more concise sentences to maintain a sense of suspense. Similarly, horror narratives might incorporate a higher frequency of ellipses and exclamation marks to enhance the feeling of suspense and intensity, whereas a non-fiction work might prioritize clarity and straightforward sentence structures. These distinctions, while perhaps not immediately obvious to human readers, can be detected with a high degree of accuracy by well-trained machine learning models.
Researchers have also found that these models, when trained on large collections of literary works, can begin to identify common tropes and themes within specific genres. This ability to recognize established patterns allows researchers to predict how likely a new piece of writing is to conform to the norms and conventions of a given genre. The application of these techniques isn't limited to straightforward classification, either. They can also provide insights into character development in relation to genre expectations, reveal hybrid genres that defy traditional categorization, and even track how genre conventions evolve over time. For example, some genres, like the detective fiction genre, have evolved considerably in terms of narrative structure, character development, and preferred endings.
There's a potential for these analytical techniques to extend beyond the usual confines of novel-length fiction. Researchers are finding that similar algorithms can be used to evaluate genre consistency in screenplays, short stories, and even poetry, expanding the scope of this kind of analysis beyond traditional prose. It's notable that some of these algorithms can even identify inconsistencies within a given genre, such as a horror story with an unexpectedly happy ending. These inconsistencies might suggest that certain aspects of the narrative could potentially confuse or alienate readers, allowing writers to refine and improve their work based on the insights provided by these computational tools. However, it's also essential to emphasize that these machine learning algorithms serve as a supplement, not a replacement, for the creativity and intuition of the human writer. The balance between human artistry and machine analysis remains a fascinating and important area for ongoing investigation.
7 Science-Backed Methods to Evaluate Writing Prompts' Effectiveness in Fiction Writing - Monitoring Reader Engagement Through Eye Movement Studies
Eye tracking studies offer a unique window into how readers engage with text, revealing the cognitive processes underlying comprehension. By monitoring eye movements, researchers can gain insights into reading patterns, including how readers process different types of language like metaphors and how they respond to emotional content. The data from these studies shows a strong connection between reader engagement and comprehension, suggesting that the deeper the engagement, the better the understanding.
Eye movement patterns, including the duration and location of fixations, can vary based on factors such as the complexity of the text and the emotional impact of the content. This adaptability in reading behavior reveals how readers adjust their approach to different types of writing. The ability to assess these adaptive strategies provides a deeper understanding of how readers navigate narratives.
In essence, these findings indicate that analyzing eye movement patterns can be a valuable tool in evaluating the effectiveness of writing prompts. By understanding how readers visually interact with text, we can gain a nuanced understanding of how they interpret narrative elements and how prompts might affect their overall experience. This knowledge can be crucial in crafting prompts that effectively encourage engagement and foster deeper comprehension of the writing.
### Monitoring Reader Engagement Through Eye Movement Studies
Eye movements offer a unique window into how readers interact with text, revealing the interplay of cognitive processes and emotional responses during comprehension. By tracking eye movements, we can gain a more nuanced understanding of how readers navigate through text, providing a direct measure of engagement and comprehension.
Eye-tracking studies have revealed that the duration of fixations—the amount of time spent focusing on a particular word or phrase—is a significant indicator of cognitive processing. Longer fixations suggest deeper processing, hinting at areas where readers grapple with understanding or experience a stronger emotional connection with the narrative. This is particularly insightful when exploring the impact of emotionally charged content in stories.
Furthermore, analyzing regression patterns—when readers' eyes backtrack to previously read text—helps identify potential trouble spots. Increased regressions can suggest confusion or difficulty with sentence structure, potentially stemming from unclear phrasing or poorly crafted writing prompts. This provides writers with a concrete way to pinpoint areas needing refinement.
Through the visualization of heat maps, which represent the intensity of reader focus, eye-tracking can illuminate which aspects of a text capture readers' attention most. This visual mapping allows writers to quickly see if elements of their writing, like the introduction of a new character or a significant plot twist, are landing with their intended impact.
Interestingly, eye movement patterns are affected by the emotional content in a text. Readers tend to dwell longer on passages with strong emotional resonance, indicating that evocative writing prompts may lead to more immersive and engaging experiences for the reader. However, these patterns are likely influenced by genre. Readers of thrillers might exhibit faster scanning due to heightened anticipation, while literary fiction might engender more leisurely and attentive reading patterns.
The latency—the delay—between encountering a compelling phrase or event and initiating an eye movement can also reveal emotional responses. A delayed reaction might indicate a moment of reflection or surprise, adding another dimension to understanding prompt efficacy.
When readers encounter a text that demands considerable cognitive effort, eye movements tend to become more erratic, with frequent and brief fixations. This suggests high cognitive load and can alert writers to passages that might need simplification or clearer explanations.
Importantly, eye tracking can help reveal the connection between reader demographics—age, experience, and other individual factors—and reading patterns. This understanding can guide writers in tailoring prompts to specific audiences, enhancing the overall impact of their work.
Perhaps the most significant benefit of eye tracking is its role as a real-time feedback mechanism. Writers can use the observed data to immediately revise and refine prompts based on reader engagement. This provides a potent feedback loop, enhancing the writing process and leading to a higher quality of storytelling.
In conclusion, eye-tracking research has shed light on the complex and dynamic interplay between eye movements and the cognitive processes that underpin reader engagement. By meticulously examining eye movement patterns, we can refine our understanding of how writing prompts influence comprehension and emotions. This knowledge empowers writers to create more engaging and effective narratives tailored to their target readers.
7 Science-Backed Methods to Evaluate Writing Prompts' Effectiveness in Fiction Writing - Calculating Literary Device Usage Frequency With Text Mining Tools
Text mining tools present a new way to examine literary works by quantifying the frequency of literary devices. Using programming languages like R, researchers can analyze the text and pinpoint how often specific words or phrases occur, helping to uncover patterns that indicate the presence of literary devices. This approach goes beyond traditional literary analysis, which relies heavily on close reading, by offering a broader perspective through methods like "distant reading" and topic modeling. Visualizing the data revealed by these methods also allows for a more intuitive understanding of the patterns within a text. Additionally, the inclusion of machine learning has broadened the scope of these tools, providing more sophisticated insights into how literary devices function within the narrative. While these tools primarily provide quantitative data on device usage, they also contribute to a richer, qualitative understanding of how authors craft their stories, offering a more holistic view of the literary art. It is important to note that human interpretation remains essential in understanding the nuance and impact of literary devices, even when aided by computational analysis.
Text analysis tools, like those used in text mining, can help us understand how often different literary devices are used in written works. For example, we can quantify how many times metaphors, similes, or alliteration occur within a collection of stories. This can provide valuable insights into how a writer's style changes over time or how they develop their stories.
It's been observed that shifts in the frequency of specific literary devices can reflect changes in a writer's core themes or their emotional tone. This information might give writers a deeper understanding of the direction their narratives are taking. We can also compare the usage of literary devices across different authors' works. This comparative analysis might highlight unique writing styles or help a writer align with specific genre conventions.
Sophisticated algorithms can even predict if a text is likely to follow established literary traditions by examining how it uses literary devices. This can be very useful for writers to get feedback on how to make their stories more coherent or artistically expressive. Some studies have suggested a connection between the frequency of certain literary devices and reader engagement. If we find that a particular device leads to more interest and better retention of the story, then writers can potentially leverage this insight for greater storytelling impact.
Text mining can also help us see how a writer's vocabulary changes when they use literary devices. Often, we see that greater diversity in language is linked to deeper emotions and more complex characters. Understanding cultural perspectives on literary devices is also important, as their impact can vary across different audiences. We might be able to determine if certain devices are better received in specific cultures through text analysis.
Furthermore, text mining can help us dissect dialogue within literary works. This helps us see how the conversational patterns and the literary devices used within the dialogue affect character development. This knowledge can be valuable for crafting more realistic and impactful dialogue in our own writing.
Machine learning is playing a growing role in the analysis of literary devices. We can train models to identify and categorize literary devices within stories and even make predictions about how changes in device usage affect the overall story. This type of predictive analytics could empower writers to refine their techniques.
Over time, we can see shifts in the use of literary devices, both within individual writers' work and across literature in general. These shifts often reflect larger changes in storytelling techniques or in what readers are seeking. By understanding these trends, we can better appreciate the dynamic nature of literary expression across different eras and cultures. While the applications of these text mining techniques in literary study are still developing, the potential for deepening our understanding of how narrative devices shape our reading experiences is exciting.
More Posts from :